How I frame data questions to make analyses more useful
A simple yet effective mental model I use to turn a vague stakeholder request into something that can be analyzed with data.
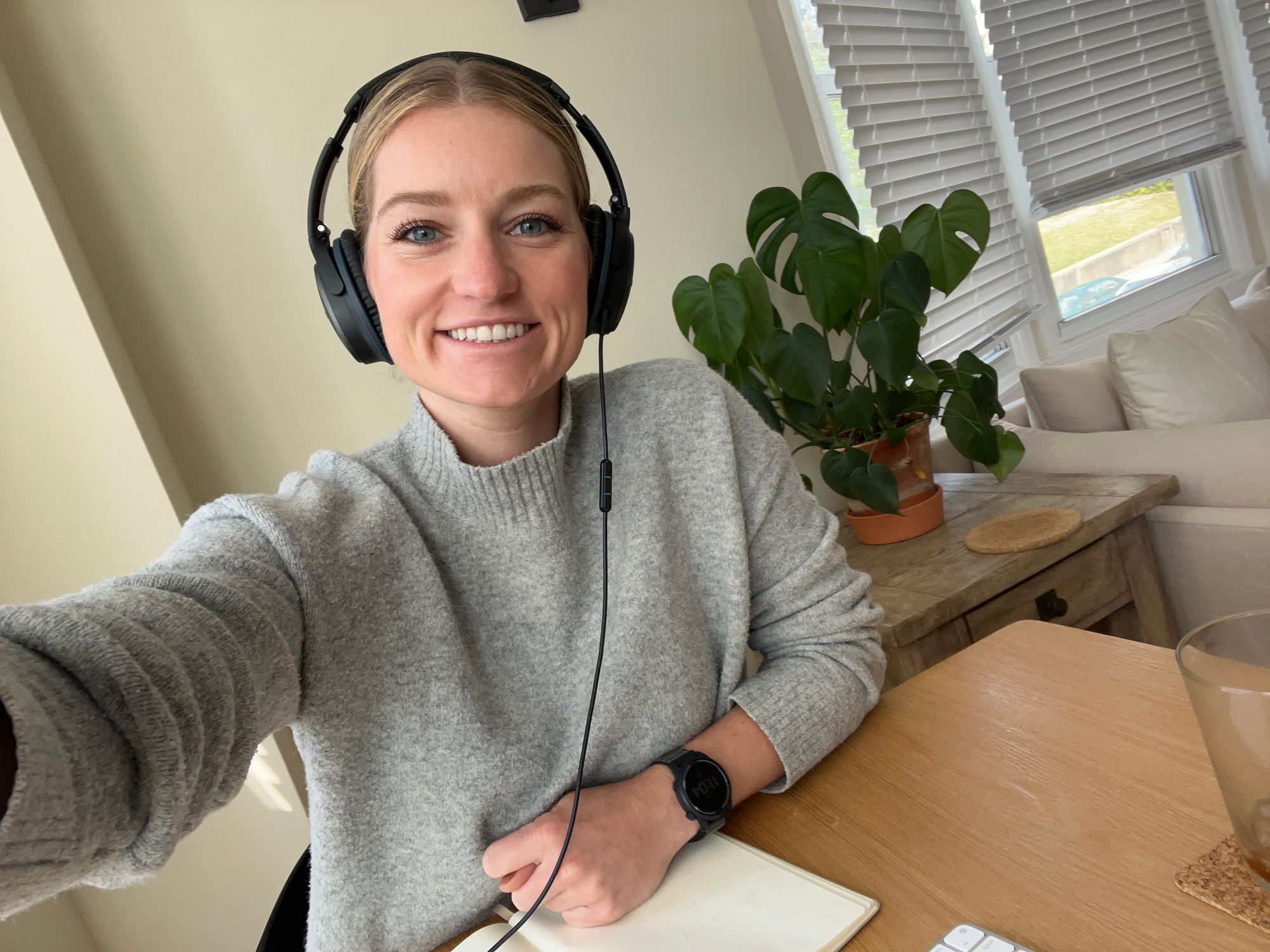
Most articles about asking or framing good data analysis questions leave you in bewilderment, buried under hundreds of the so-called “good questions” and checklists for asking them. In this post, I want to suggest one simple and effective model that handled about 80% of the questions I’ve dealt with in the past 3.5y as the Head of Data at Narrator. I call it “lever-KPI.”
The idea is to frame the (initially vague) data questions you get from stakeholders this way: How does [lever] impact [KPI]?
For example:
- How does gender impact web conversion rate?
- How does length of the first workout class influence likelihood to take another class?
- How does price influence churn rate?
- How does opening an email influence likelihood to purchase?
As you may have already guessed, “lever” is the thing you think has an influence on the KPI you’re trying to optimize. I like imagining it as a physical lever our team can push to move the KPI forward! As the famous Greek said, if it’s long enough, we shall move the world. (Unlike in Archimedes’s business of moving the world, though, in data, you usually find many small levers rather than a single giant one.)
The “KPI” part is kind of self-explanatory. It is the metric you’re working towards, such as “retention rate” or “average order value.”
In many years of applying this model, I’ve discovered several benefits of framing questions this way:
- Changes your thinking. After some time, the model changed my perception. Like writers who see an interesting subject and a theme where others see nothing (subject-theme is a model just like lever-KPI), I started seeing those levers and KPIs everywhere I went as if I got some X-ray goggles on my head. The model also improved my work speed. Now I spot those levers and KPIs in otherwise vague questions much faster than ever before.
- Ensures an actionable insight. The model helps to avoid wasting time exploring interesting but useless data. For example, I once did a clustering analysis on website users. The one thing that I found was that we had a small amount of bots on the site, and they acted very differently than the rest of the visitors. That was really interesting, but not really useful. If I had the model in mind, I’d not waste time on those bots.
- Makes analyses simpler. Having a model with two variables and a relationship between them saves you from the problem of numerous confounds that can mask the importance of a particular variable when you’re doing something really complex. No confounds = no need to remove them individually to test the significance of each factor.
- Supports good hypotheses. When thinking about data, people tend to think in business metrics and plots. However, you are much more likely to find an actionable insight, something that actually influences your KPI, that you can change, if you think about things on a human level. For example, instead of an analysis question that says, “let’s understand how total promotional emails correlates to a higher sign-up rate,” the lever-KPI model requires you to say, “will Joe be more likely to do something (KPI) if he receives this promotional email (KPI).” Framed this way, the question is more likely to yield an actionable insight.
- Aids in stakeholder communication. Many stakeholders have a very primitive model of what actually happens in data (akin to a car: “pedal to the metal, and it goes”), expertise in a different field/role (and therefore interests in high-level things like revenue, growth, etc.), and often don’t know what data is available. Because of that, they tend to ask very broad or aimless questions that can be very hard or even impossible to analyze with data. The lever-KPI model solves this problem because you must identify a specific attribute/behavior of a customer and their goal state to apply it. It’s like a pre-flight protocol the pilot commences to ensure he does not forget anything obvious.
- Helps to avoid analysis paralysis. The model is deliberately narrow, which means you will not be boiling the ocean trying to figure out “what are the drivers of churn?” Those super broad questions usually lead straight into analysis paralysis, and the lever-KPI model prevents you from (gently) going into that good night.
Hopefully, you’ll get to experience some of the lever-KPI model benefits yourself by using it!